The majority of times I see people sharing information about their A/B tests, it looks something like:
“I just ran a test and got 40 visits at a 12.5% conversion rate on page A whereas I got 7 visits at a 14% conversion rate on page B, so Page B is clearly better!”
This is wrong.
But it’s wrong in the most insidious way: being wrong with data. It feels like when you have hard numbers that support a statement, you’re sure to feel extra confident when you’re wrong.
There are two reasons for this.
One is you need to be sure of the confidence interval of your results, and the other is that you need to make sure you’re testing a substantial enough change that a performance change is gonna be more than sheer chance.
Let’s dive in about this.
Confidence Interval
Confidence interval is how likely it is that there is a statistical difference between two different averages.
Look at it this way. With the above numbers I used, you have two landing pages.
Page A had 40 visits at a 12.5 conversion rate.
$40*.125=5$
So there were 5 conversions.
Page B had 7 visits at a 14% conversion rate.
$7*.14=1$
There was just 1 conversion.
The average conversion rate
for these two pages was 12.5% and 14%, respectively. Given the size of each landing page’s audience and the averages they produced, how likely is it that this difference is a random chance? How confident can you be that the interval (the space between the two averages) is a result of an actual difference in performance?
There’s a formula for this. If you recall your days of college algebra, you can probably suss it out.
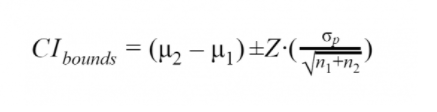
You can read up what all of the above variables mean in this two-sided test equation at the link above. Indeed, if you’re conducting a lot of high-stakes A/B tests with percentages, you should probably avoid confidence interval equations entirely as they’re slightly inaccurate compared to more modern (even more complex!) formulas.
But this isn’t a maths blog, so let’s instead focus on what we should do instead of going to Wolfram Alpha and researching the formula in depth.
How to understand the relevance of your test result
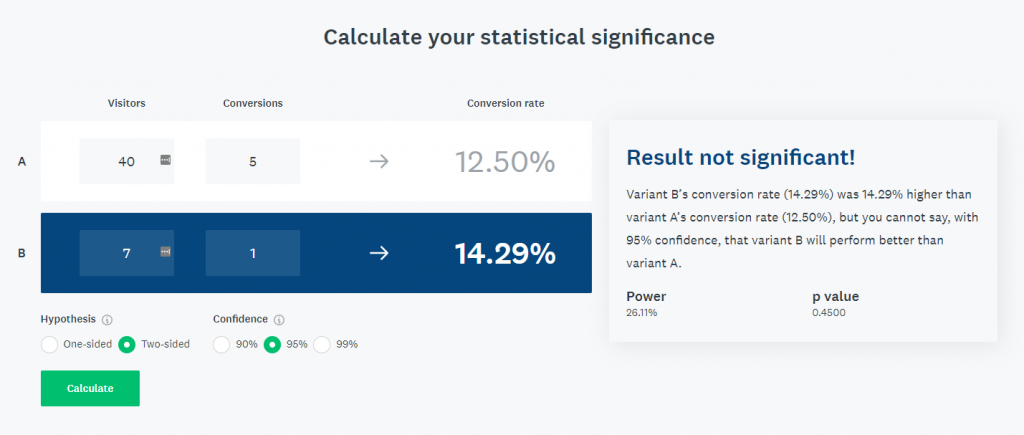
In short, the difference in performance is too small to have much confidence in. I don’t just say this. The handy A/B tests calculator at Survey Monkey does, too.
Whenever you run a test of any size, it’s very helpful to check with a simple trip to a calculator like this one to be sure that your results are relevant.
There are two things worth noting here: 1 is even with a 95% confidence interval, you’re still going to get incorrect results 1 time in 20 or so. And 2, if you’re not testing something that’s ambitious enough, you’ll rarely get unambiguous results.
For your A/B tests, test big ideas:
Recently I was on a consulting call with a potential client and, with some changes to protect the innocent, here are the two headlines that they had most recently tested for their company.
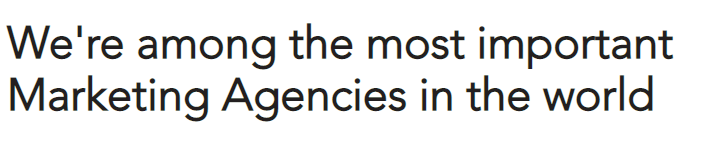

I think they are both pretty bad headlines, but I also think that there is virtually 0 chance that there will be a large difference in performance between the two.
To contrast to that, here are some ads I tried out for a client back at the beginning of the year.
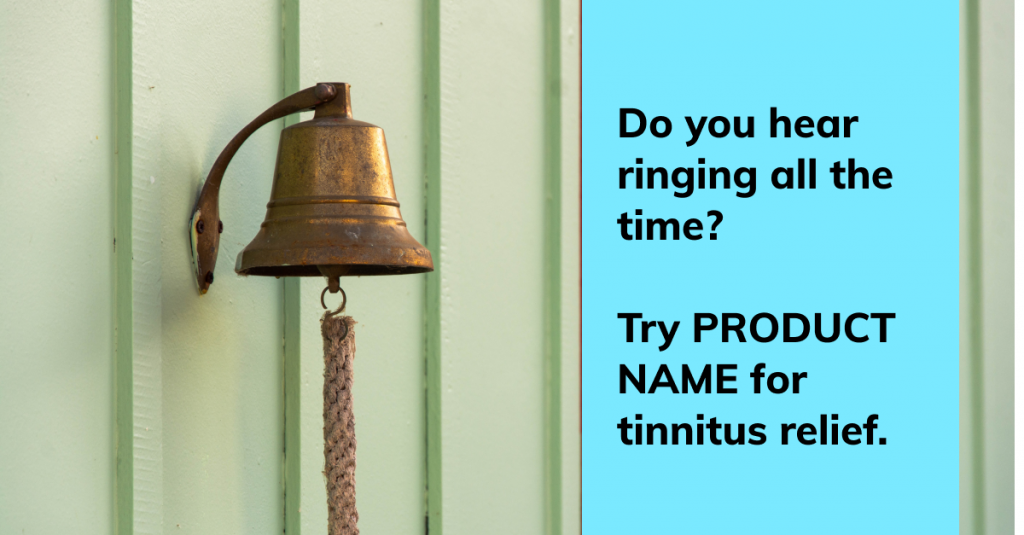
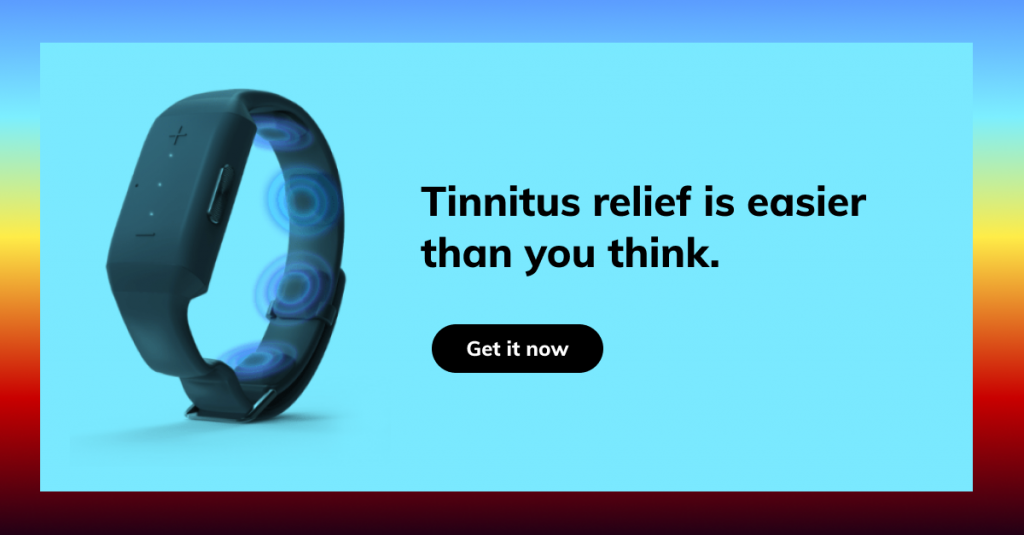
Say what you will about the design of the ads—this was before I’d hired on Ram, our in-house design master—but there’s no question that these are two very different ways to sell an identical benefit.
Quit running bad experiments!
— CrowdTamers (@CrowdTamers) October 12, 2021
When you’re doing your very first audience tests as part of a grow-to-market campaign, don’t handicap yourself with a small vision.
Listen to the audiogram to know more. pic.twitter.com/qMkSyoHglR
And this is the crux of the issue. Many people think that A/B tests mean making a minor change to their headline or different button color. There are times and places where that’s adequate to the needs of a very refined and finalized product and landing page design, but if you’re getting a new idea off the ground and you want to launch something today, you need to swing big.
Which landing page is better?
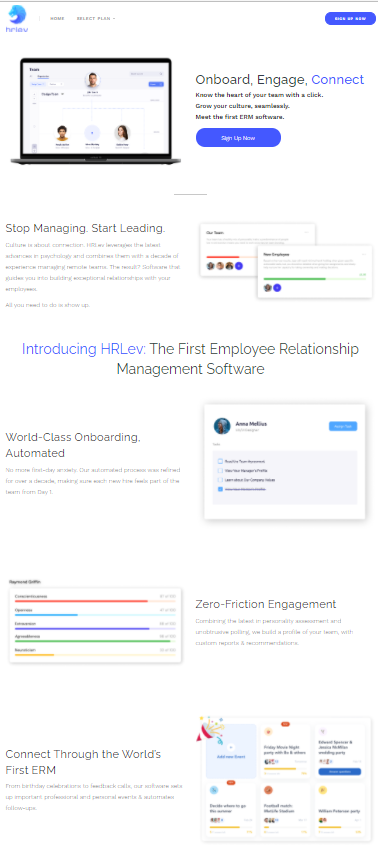
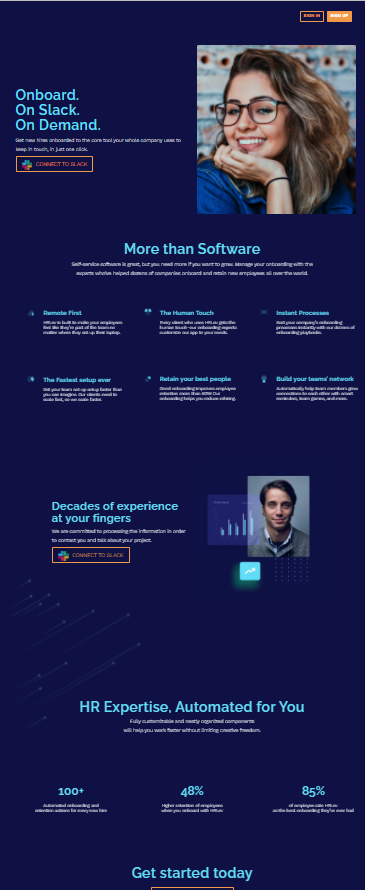
Before we ran the test, I didn’t know—and neither did the client. But you can believe that with such different approaches, I was confident that once the test was done I’d have a relevant result to share. And I did!
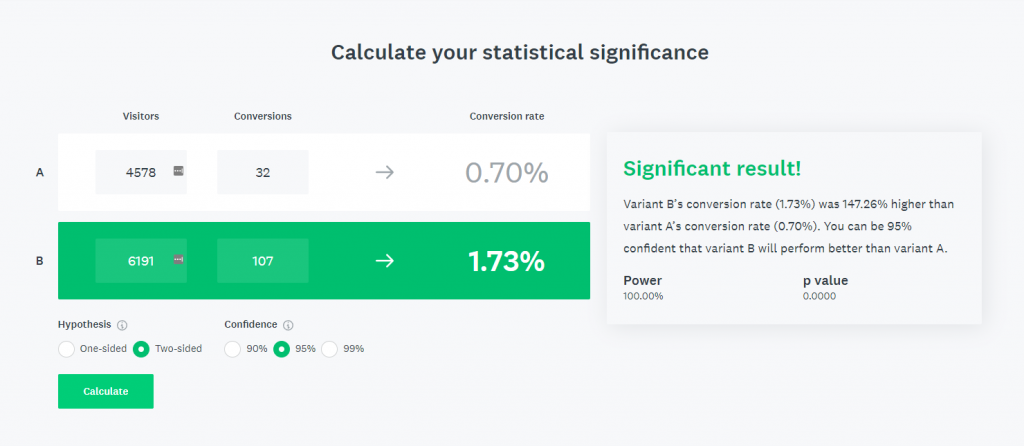
So, what tests are you running today? I’d love to hear about them @trevorlongino on Twitter or @crowdtamers. Let me know!